Simple example code on hyperparameter optimization for DNN regression models.
Table of Contents
Prepare data
import numpy as np
import pandas as pd
from sklearn.model_selection import train_test_split
from sklearn.preprocessing import StandardScaler
# create range of monthly dates
download_dates = pd.date_range(start='2019-01-01', end='2020-01-01', freq='MS')
# URL from Chrome DevTools Console
base_url = ("https://climate.weather.gc.ca/climate_data/bulk_data_e.html?format=csv&"
"stationID=51442&Year={}&Month={}&Day=7&timeframe=1&submit=Download+Data") # add format option to year and month
# create list of remote URL from base URL
list_of_url = [base_url.format(date.year, date.month) for date in download_dates]
# download and combine multiple files into one DataFrame
df = pd.concat((pd.read_csv(url) for url in list_of_url))
data = df.loc[:, (df != df.iloc[0]).any()]
data=data[data.columns[data.isnull().mean() < 0.9]]
keepcolumns=['Temp (°C)','Dew Point Temp (°C)', 'Rel Hum (%)','Stn Press (kPa)']
data=data[keepcolumns]
predictors=['Temp (°C)','Dew Point Temp (°C)', 'Stn Press (kPa)']
predictand=['Rel Hum (%)']
X = data[predictors].values
y = data[predictand].values
X_train, X_test, y_train, y_test = train_test_split(X, y, test_size=0.30, random_state=40)
transformer = StandardScaler().fit(X_train)
X_train_t=transformer.transform(X_train)
X_test_t =transformer.transform(X_test)
ytransformer = StandardScaler().fit(y_train)
y_train_t=ytransformer.transform(y_train)
y_test_t =ytransformer.transform(y_test)
Example 1: search for optimal units
Build model
from sklearn.metrics import mean_squared_error
from math import sqrt
import keras
from keras.models import Sequential
from keras.layers import Dense
import keras_tuner
def build_model(hp):
model = keras.Sequential()
model.add(keras.layers.Dense(
hp.Choice('units', [8, 16, 32]),
activation='relu'))
model.add(keras.layers.Dense(
hp.Choice('units', [4,8,16]),
activation='relu'))
model.add(keras.layers.Dense(
hp.Choice('units', [1,4,8]),
activation='relu'))
model.add(keras.layers.Dense(1, activation="softmax"))
model.compile(loss='mse')
return model
Search the best hyperparameters
tuner = keras_tuner.RandomSearch(
build_model,
objective='val_loss',
overwrite = True,
max_trials=5)
tuner.search(x_train_t, y_train_t, epochs=5, validation_data=(x_test_t, y_test_t))
best_model = tuner.get_best_models()[0]
best_model.build(input_shape=(None, 3))
best_model.summary()
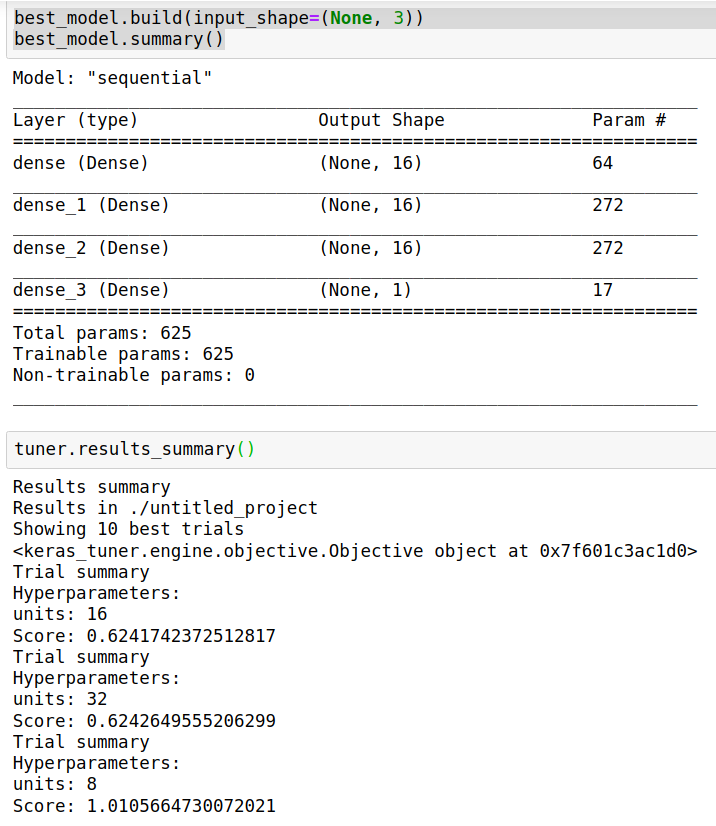
# Get the top 5 hyperparameters.
best_hps = tuner.get_best_hyperparameters(5)
# Build the model with the best hp.
model = build_model(best_hps[0])
# Fit with the entire dataset.
x_all = np.concatenate((X_train_t, X_test_t))
y_all = np.concatenate((y_train_t, y_test_t))
model.fit(x=x_all, y=y_all, epochs=10)
pred_train= model.predict(X_train_t)
print(np.sqrt(mean_squared_error(y_train_t,pred_train)))
pred= model.predict(X_test_t)
print(np.sqrt(mean_squared_error(y_test_t,pred)))
0.793593549087112
0.7900202612742904
Example 2: search for optimal units and activation function
from sklearn.metrics import mean_squared_error
from math import sqrt
import keras
from keras.models import Sequential
from keras.layers import Dense
import keras_tuner
def build_model(hp):
model = keras.Sequential()
for i in range(hp.Int("num_layers", 1, 3)):
model.add(
layers.Dense(
# Tune number of units separately.
units=hp.Int(f"units_{i}", min_value=4, max_value=32, step=4),
activation=hp.Choice("activation", ["relu", "tanh"]),
)
)
model.add(keras.layers.Dense(1, activation="softmax"))
model.compile(loss='mse')
return model
tuner = keras_tuner.RandomSearch(
build_model,
objective='val_loss',
overwrite = True,
max_trials=5)
tuner.search(X_train_t, y_train_t, epochs=5, validation_data=(X_test_t, y_test_t))
best_model = tuner.get_best_models()[0]
# Get the top 5 hyperparameters.
best_hps = tuner.get_best_hyperparameters(5)
# Build the model with the best hp.
model = build_model(best_hps[0])
# Fit with the entire dataset.
x_all = np.concatenate((X_train_t, X_test_t))
y_all = np.concatenate((y_train_t, y_test_t))
model.fit(x=x_all, y=y_all, epochs=10)
pred_train= model.predict(X_train_t)
print(np.sqrt(mean_squared_error(y_train_t,pred_train)))
pred= model.predict(X_test_t)
print(np.sqrt(mean_squared_error(y_test_t,pred)))
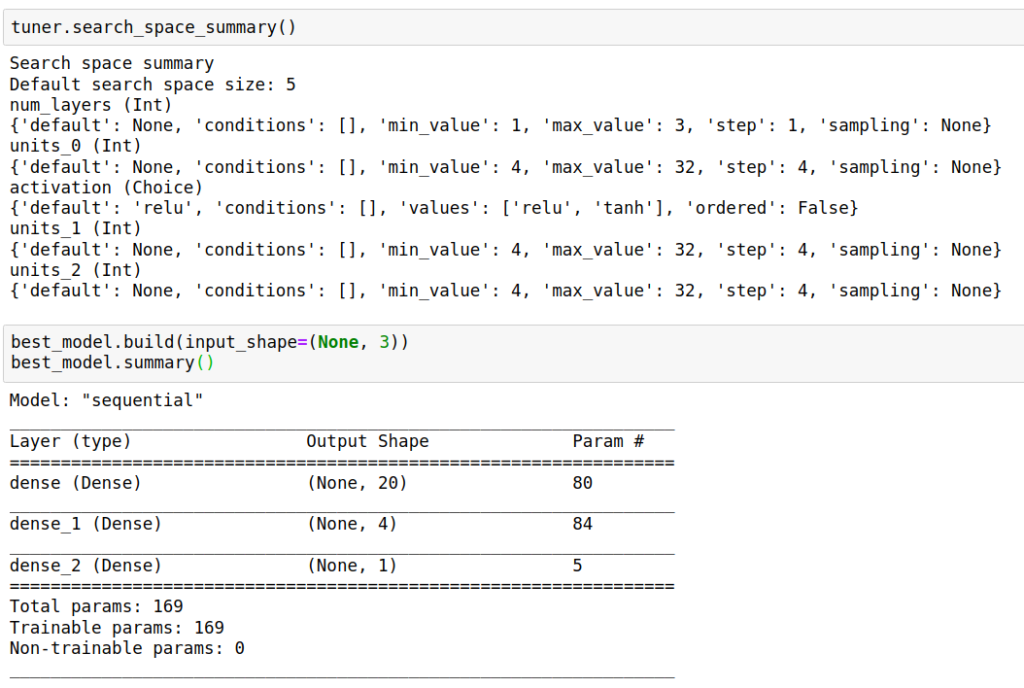
Example 3: use AutoKeras to automatically find the best model
Simple example
import autokeras as ak
# It tries 10 different models.
reg = ak.StructuredDataRegressor(max_trials=3, overwrite=True)
# Feed the structured data regressor with training data.
reg.fit(X_train_t, y_train_t, epochs=10)
# Predict with the best model.
predicted_y = reg.predict(X_test_t)
# Evaluate the best model with testing data.
print(reg.evaluate(X_test_t, y_test_t))
model = reg.export_model()
model.summary()

Customized Search Space
import autokeras as ak
input_node = ak.StructuredDataInput()
output_node = ak.StructuredDataBlock(categorical_encoding=False)(input_node)
output_node = ak.RegressionHead()(output_node)
reg = ak.AutoModel(
inputs=input_node, outputs=output_node, overwrite=True, max_trials=3
)
reg.fit(X_train_t, y_train, epochs=10)
# Predict with the best model.
predicted_y = reg.predict(X_test_t)
# Evaluate the best model with testing data.
print(reg.evaluate(X_test_t, y_test_t))
model = reg.export_model()
model.summary()
Note: Data should be split into train, validation and test parts for tuning. As an example, here we just use test part as validation part.
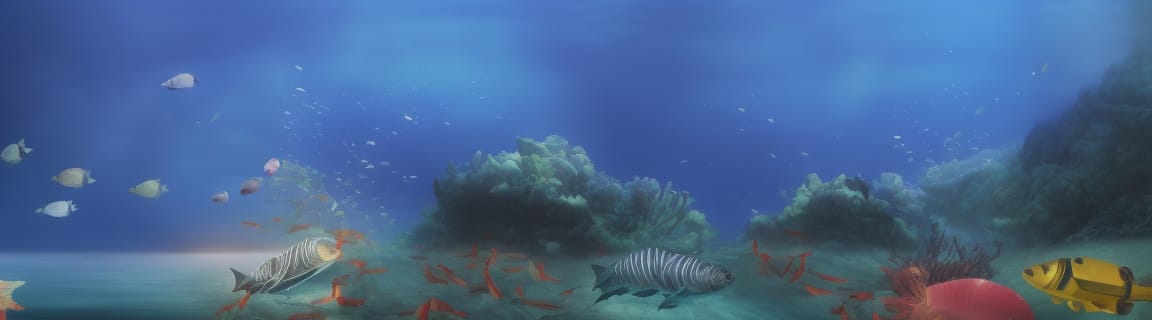